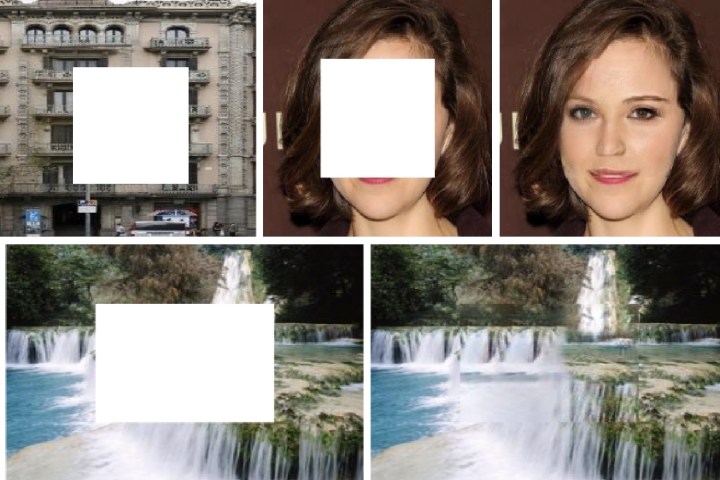
You only need to go check out the latest Hollywood blockbuster or pick up a new AAA game title to be reminded that computer graphics can be used to create some dazzling otherworldly images when called for. But some of the most impressive examples of machine-generated images aren’t necessarily alien landscapes or giant monsters, they’re image modifications that we don’t even notice.
That’s the case with a new A.I. demonstration created by computer scientists in China. A collaboration between Sun Yat-sen University in Guangzhou and Beijing’s Microsoft Research lab, they’ve developed a smart artificial intelligence which can be used to accurately fill in blank areas in an image: Whether that’s a missing face or the front of a building.
Called inpainting, the technique uses deep learning technology to fill these spaces either by copying image patches on the remainder of the picture, or by generating new areas that look convincingly accurate. The tool, which is referred to by its creators as PEN-Net (Pyramid-context ENcoder Network), does this image restoration by “encoding contextual semantics from full-resolution input and decoding the learned semantic features back into images.” The resulting Attention Transfer Network (ATN) images are not only impressively realistic, but the tool is also very quick to learn.
“[In this work, we proposed] a deep generative model for high-quality image inpainting tasks,” Yanhong Zeng, a lead author on the project, who is associated with both Sun Yat-sen University’s School of Data and Computer Science and Key Laboratory of Machine Intelligence and Advanced Computing, told Digital Trends. “Our model fills missing regions from deep to shallow at all levels, based on a cross-layer attention mechanism, so that both structure and texture coherence can be ensured in inpainting results. We are excited to see that our model is capable of generating clearer textures and more reasonable structures than previous works.”
As Zeng notes, this isn’t the first time researchers have developed tools to carry out inpainting. However, the team’s PEN-Net system demonstrates impressive results next to classical method PatchMatch and even other state-of-the-art approaches.
“Image inpainting has a wide range of applications in our daily life,” Zeng continued. “We are now planning to apply our technology in image editing — especially for object removal [and] old photo restoration.”
A paper describing the work, titled “Learning Pyramid-Context Encoder Network for High-Quality Image Inpainting,” is available to read on preprint paper repository Arxiv.
Editors' Recommendations
- New ‘poisoning’ tool spells trouble for AI text-to-image tech
- This new Photoshop tool could bring AI magic to your images
- I pitched my ridiculous startup idea to a robot VC
- Zoom’s A.I. tech to detect emotion during calls upsets critics
- Analog A.I.? It sounds crazy, but it might be the future