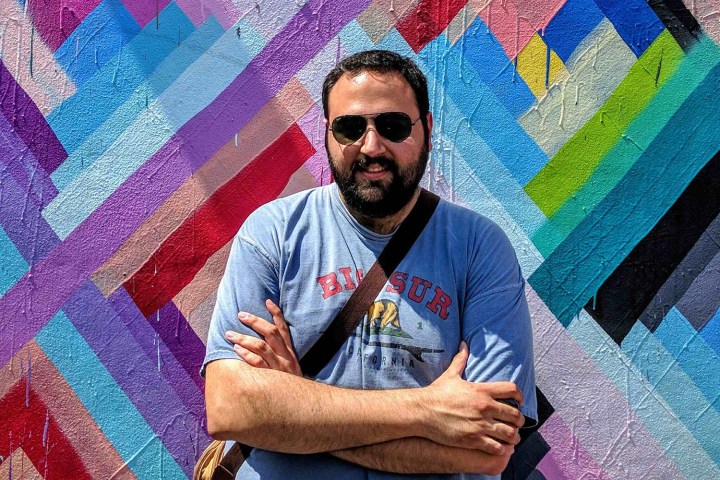
When Snapchat was first pitched as part of a Stanford mechanical engineering class, the course’s horrified teaching assistant openly wondered if the app’s creators had built a sexting app. Less than a decade later, Snapchat could help solve one of the biggest problems currently facing tech: stopping the spread of “fake news” online.
With this goal in mind, Snap Research — the research division of Snap, Inc. — recently donated funding to a University of California, Riverside project, aiming to find a new way of detecting fake news stories online. The algorithm UC Riverside has developed is reportedly capable of detecting fake news stories with an impressive accuracy level of up to 75 percent. With Snap’s support, they hope to further improve this.
“As I understand it, they’re very interested in having a good grasp on how one could understand this problem — and solve it ultimately.”
“Snap is not one of the first companies that would come to mind given [this problem],” Vagelis Papalexakis, Assistant Professor in the Computer Science & Engineering Department at UC Riverside, told Digital Trends. “Nevertheless, Snap is a company which handles content. As I understand it, they’re very interested in having a good grasp on how one could understand this problem — and solve it ultimately.”
What makes UC Riverside’s research different to the dozens, maybe even hundreds, of other research projects trying to break the fake news cycle is the ambition of the project. It’s not a simple keyword blocker, nor does it aim to put a blanket ban on certain URLS. Nor, perhaps most interestingly, is it particularly interested in the facts contained in stories. This makes it distinct from fact-checking websites like Snopes, which rely on human input and evaluation instead of true automation.
“I do not really trust human annotations,” Papalexakis said. “Not because I don’t trust humans, but become this is an inherently hard problem to get a definitive answer for. Our motivation for this comes from asking how much we can do by looking at the data alone, and whether we can use as little human annotation as possible — if any at all.”
The signal for fake news?
The new algorithm looks at as many “signals” as possible from a news story, and uses this to try and classify the article’s trustworthiness. Papalexakis said: “Who shared the article? What hashtags did they use? Who wrote it? Which news organization is it from? What does the webpage look like? We’re trying to figure out which factors [matter] and how much influence they have.”
For example, the hashtag #LockHerUp may not necessarily confirm an article is fake news by itself. However, if a person adds this suffix when they share an article on Twitter, it could suggest a certain slant to the story. Add enough of these clues together, and the idea is that the separate pieces add up to a revealing whole. To put it another way, if it walks like a duck and quacks like a duck, chances are that it’s a duck. Or, in this case, a waddling, quacking, alt-right Russian duck bot.
“Our interest is to understand what happens early on, and how we can flag something at the early stages before it starts ‘infecting’ the network,” Papalexakis continued. “That’s our interest for now: working out what we can squeeze out of the contents and the context of a particular article.”
The algorithm developed by Papalexakis’ group uses something called tensor decomposition to analyze the various streams of information about a news article. Tensors are multi-dimensional cubes, useful for modeling and analyzing data which have lots of different components. Tensor decomposition makes it possible to discover patterns in data by breaking a tensor into elementary pieces of information, representing a particular pattern or topic.
“Even a ridiculously small number of annotated articles can lead us to really, really high levels of accuracy”
The algorithm first uses tensor decomposition to represent data in such a way that it groups possible fake news stories together. A second tier of the algorithm then connects articles which are considered to be close together. Mapping the connection between these articles relies on a principle called “guilt by association,” suggesting that connections between two articles means they are more likely to be similar to one another.
After this, machine learning is applied to the graphs. This “semi-supervised” approach uses a small number of articles which have been categorized by users, and then applies this knowledge to a much larger data set. While this still involves humans at some level, it involves less human annotation than most alternate methods of classifying potential fake news. The 75 percent accuracy level touted by the researchers is based on correctly filtering two public datasets and an additional collection of 63,000 news articles.
“Even a ridiculously small number of annotated articles can lead us to really, really high levels of accuracy,” Papalexakis said. “Much higher than having a system where we tried to capture individual features, like linguistics, or other things people may view as misinformative.”
A cat-and-mouse game for the ages
From a computer science perspective, it’s easy to see why this work would appeal to Vagelis Papalexakis and the other researchers at UC Riverside — as well as the folks at Snapchat. Being able to not only sort fake news from real news, but also distinguish biased op-eds from serious journalism or satirical articles from The Onion is the kind of big data conundrum engineers dream of.
The bigger question, however, is how this algorithm will be used — and whether it can ultimately help crack down on the phenomenon of fake news.
Snap’s contribution to the project (which amounts to a $7,000 “gift” and additional non-financial support) does not guarantee that the company will adopt the technology in a commercial product. But Papalexakis said he hopes the research will eventually “lead to some tech transfer to the platform.”
The eventual goal, he explained, is to develop a system that’s capable of providing any article with what amounts to a trustworthiness score. In theory, such a score could be used to filter out fake news before it even has the chance to be glimpsed by the user.
This is a not dissimilar idea to machine learning email spam filters, which also apply a scoring system based on factors like the ratio of image to text in the body of a message. However, Papalexakis suggested that a preferable approach might be simply alerting users to those stories which score high in the possible fake category — “and then let the user decide what to do with it.”
One good reason for this is the fact that news does not always divide so neatly into spam vs. ham categories, as email does. Sure, some articles may be out-and-out fabrication, but others may be more questionable: featuring no direct lies, but nonetheless intended to lead the reader in one certain direction. Removing these articles, even when we might find opinions clashing with our own, gets into stickier territory.
“This falls into a gray area,” Papalexakis continued. “It’s fine if we can categorize this as a heavily biased article. There are different categories for what we might call misinformation. [A heavily biased article] might not be as bad as a straight-up false article, but it’s still selling a particular viewpoint to the reader. It’s more nuanced than fake vs. not fake.”
Ultimately, despite Papalexakis’ desire to come up with a system that uses as little oversight as possible, he acknowledges that this is a challenge which will have to include both humans and machines.
“I see it as a cat-and-mouse game from a technological point of view,” he said. “I do not think that saying ‘solving it’ is the right way to look at it. Providing people with a tool that can help them understand particular things about an article is part of the solution. This solution would be tools that can help you judge things for yourself, staying educated as an active citizen, understanding things, and reading between the lines. I don’t think that a solely technological solution can be applied to this problem because so much of it depends on people and how they see things.”