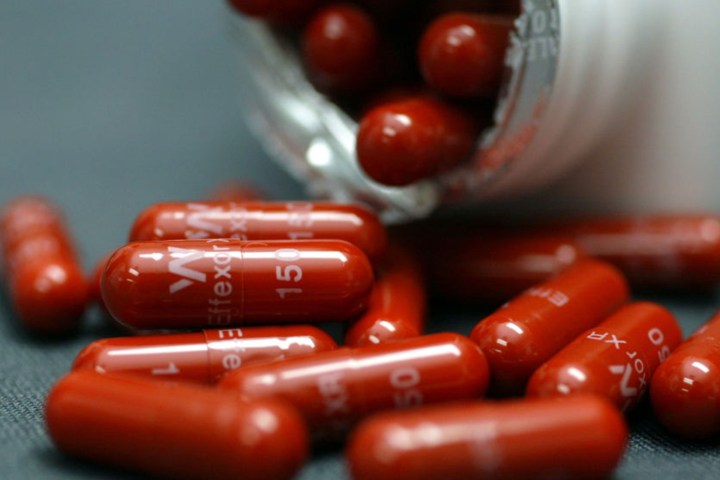
Between the fake news potential of deepfakes, the fear of robots stealing jobs, and the occasional call for automated systems to have control of the nuclear button, A.I.’s public image could do with a PR makeover here in 2019. Could saving a few million lives help?
That’s something a new biotech pharmaceutical startup called Insilico Medicine may be able to help with. Combining genomics, big data analysis, and deep learning, the company — which is based in Rockville in Johns Hopkins University’s Emerging Technology Centers — has been using artificial intelligence algorithms to potentially discover the next world-changing drug. Using two of the most exciting and popular A.I. techniques of the moment, it’s found a way of discovering drug molecules not only far more cheaply than usual, but also much, much, much faster.
“There is a new concept in artificial intelligence called Generative Adversarial Networks (GANs), which was first introduced in 2014,” Alex Zhavoronkov, CEO of Insilico, told Digital Trends. “Since then, it has been applied to [the] generation of novel images, text, and even music. The worst application seen to date were the deepfakes.”
Two promising approaches
A Generative Adversarial Network consists of two distinct neural networks: a generator and a discriminator. The two play the part of what we might call “frenemies,” simultaneously competitors and cooperators. The role of the generator is to create fake signals or data that’s able to fool the discriminator. The discriminator, meanwhile, is there to try and spot the difference between real and fake signals. In the same way that competition between rivals can ultimately push both to new peaks in performance, the Generative Adversarial Network produces better and better results as the generator seeks to outdo the discriminator. Eventually, the discriminator can no longer tell the difference between what is real and what is fake; leaving the generator to create new signals of unparalleled quality.
Since 2016, Insilico Medicine researchers have been working to get GANs to “imagine” new molecules with drug-like properties. In 2017, they combined this with another type of groundbreaking A.I. in the form of Reinforcement Learning. Reinforcement Learning, most famously used by Google DeepMind to produce a video game-playing A.I., is built around the notion of A.I. agents which use trial-and-error to maximize some kind of reward. If a Generative Adversarial Network is your laid-back artistic friend, gradually honing its techniques, then Reinforcement Learning is your hyper-competitive buddy who can turn just about anything into a winnable competition.
Insilico set out to use this combination of A.I. approaches to generate novel molecules for a known fibrosis (and possibly cancer) target called DDR1. “This process usually takes a couple years and is very expensive,” Zhavoronkov said. “But [in our latest paper], the A.I. managed to do this in 21 days. The ‘imagined’ molecules were then synthesized and tested in many experiments, including in mice.”
Training its A.I.
Previously, in order to find a small molecule for a specific protein target it was necessary to test hundreds of thousands, or possibly even millions, of molecules. Drug discovery is notoriously resource intensive, with timelines that measure in the decades, and costs which can reach as high as $2.6 billion for a single new drug. Zhavoronkov invokes the old cliche of finding a new functioning drug molecule as being akin to searching for a needle in a haystack. With the team’s A.I. approach, however, this paradigm is, as technologists are want to say, shifted. It allows the team to “generate perfect needles” with specified properties.
In the 21 day timespan described in the team’s Nature Biotechnology paper, the A.I. was able to create 30,000 designs for molecules targeting the specified protein. Six of these were then synthesized in the lab and the most promising one tested successfully in mice. The total process took 46 days.
Insilico calls its drug design machine learning system GENTRL, short for Generative Tensorial Reinforcement Learning. “We trained GENTRL on the entire chemical space and the molecules are already known to work on DDR1 kinase,” he said. “There are a few available. Think of it as training ‘imaginative’ A.I. on all human faces, and then showing it a few pictures of Brad Pitt and asking it to imagine pictures of someone who looks like Brad Pitt but 15 years younger with blue eyes and female. It will have some [of the] original properties, but will look very different and will have new properties. We did something similar with molecules.”
What’s next for the research?
This isn’t the only A.I. startup that’s using a similar approach to drug discovery. IBM Watson has explored the use of machine intelligence to help develop drugs, although it’s since pulled back on this. Other research institutes such as the U.K.’s University of Manchester have also developed “robot scientists” for helping automate the process of drug discovery.
While there’s still more work to be done before the resulting A.I.-designed drugs can be sold to patients, Insilico’s work is nonetheless promising research. By making the development process of drugs cheaper, it could result in the end consumer prices being reduced. If companies do not need to reap such massive profits on new drugs they develop, it could also mean that it is more economically viable to develop drugs for certain tropical diseases.
“This approach when integrated into the automated pipelines for drug discovery, that can work on multiple target classes, should be able to cut about 1-2 years off the pharma R&D cycle,” Zhavoronkov said. “[It will additionally help] quickly validate the targets using novel chemistry. We also expect the molecules to be better and safer.”